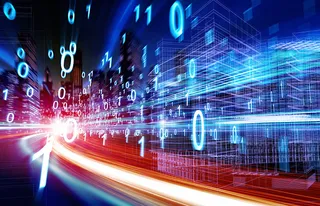
The future of precision medicine part 2: data is king
In the first part of this series on the challenges and opportunities faced by precision medicine, we discussed the capabilities and limitations of precision medicine as we currently understand it.
This second instalment will focus on the key topic of data, asking and answering the questions of what data is needed, how it is used, what should it look like and what concerns this raises for patients and society.
It is fair to say that data and data science are, and will continue to be, the great enablers of much of what precision medicine aims to achieve, alongside parallel advances in our understanding of the genetic and environmental bases of disease.
Set against the promise of the field is the acute awareness among industry experts, researchers and clinicians that, above all, precision medicine relies on data that is high in quantity, quality and diversity.
Quantity of data
A large quantity of data is required for the sufficient statistical powering of analyses to enable statistically significant observations to be made about (for example) the efficacy of a treatment in a given population, or the risk of a particular adverse effect.
The more data points that are available, the more reliably we are able to make observations based on that data, and the more likely it is that smaller signals in the data may be detected.
This is particularly the case for the detection of rare and ultra-rare genetic variations and/or diseases, which may occur in a tiny proportion of the overall population but have drastic and devastating consequences for that group.
The difficulty in compiling sufficient data on rare diseases and mutations is one of the reasons for the initial focus of the Genomics England 100,000 Genomes Project on cancer and rare disease patients.
Quality of data
In this context, quality of data means more than just the reliability of data collection processes or of individual data points (important as that is) but also entails the comprehensiveness of the data profile of individual patients.
When talking about precision medicine there is often a strong tendency to focus on genomic data. This is understandable, but unnecessarily limiting; as Sir John Chisholm, executive chair of Genomics England noted in his Westminster Health Forum (WHF) address in December last year, it has been observed (in a US context) that health is 30% genetic, 60% environmental and 10% influenced by healthcare systems.
Accordingly, in its fully realised form, precision medicine must not be blinkered by an overemphasis on genetics, but will require a broader set of patient information, including (for example) information on environmental factors, medical history, phenotypic data and microbiome data.
This broader approach to the collection of relevant patient data is consistent with the reality that the determinants of health outcomes are not limited to medico-scientific factors such as a patient’s genetics or biology, but include social and environmental factors such as geography, racial self-identification and socio-economic status.
Diversity of data
Diversity of data means collecting data from a wide cross-section of genetic and demographic backgrounds to form a rich and inclusive dataset in which every part of society is represented.
At present, the majority of genetic information that has been generated by scientific research concerns Caucasian populations.
Speaking at the World Economic Forum (WEF), Tan Chorh Chuan, executive director of Singapore’s Office for Healthcare Transformation, noted that in 2016 a survey of 2,511 genome-wide association studies (corresponding to nearly 35 million samples) found that only 19% of participants were of non-European descent (and, of that non-European 19%, the majority were Asian).
He observed that, in underrepresented genetic populations, there is a risk that genetic markers might be wrongly assigned to disease, such that the implementation of precision medicine for one group in fact results in “imprecise medicine” for another.
This is no phantom risk; it has been reported that multiple patients of African ancestry have been misdiagnosed as possessing genetic variants associated with hypertrophic cardiomyopathy, an error stemming from lack of diversity in the control groups for the studies that mistakenly identified those variants as pathogenic.
The present lack of diversity in the genetic data that has been collected and analysed increases the risk of Euro-centric bias in diagnosis and treatment and represents a glaring gap in our current understanding.
This is a clear equality issue that needs to be redressed by larger sets of more diverse patient information in order to be confident of the predictive power of biomarkers in different populations and avoid creating or widening a genetic equality gap, with real potential consequences for quality of life and life expectancy.
Collecting and unlocking the data
The collection of such a quantity and range of datasets is a Herculean task, which requires significant investment and additionally poses difficult issues in terms of consistency of methodology within and across different initiatives.
Speaking at the 2018 annual meeting of WEF in Davos, Switzerland, Jay Flatley, executive chairman of gene sequencing company Illumina, said that it is up to publicly-funded “big science” population genomics initiatives like the 100,000 Genomes Project to generate this data; and even once the data has been collected there will be a challenge to work out how that data can be shared and pooled to increase the power of those datasets (from practical and legal/regulatory perspectives).
Looking beyond initiatives to generate genomic patient data, vast stores of patient history and phenotypic data captured in the form of existing patient records represent an immense untapped resource for precision medicine initiatives.
Countries which have a centralised healthcare system and electronic recording of healthcare records (such as the NHS in the UK, and the respective Medicare systems in Canada and Australia) are at a relative advantage in this respect.
However, the difficulties in accessing and making sense of such records, even for the patients they belong to, are well documented. The usefulness of the legacy patient datasets we currently possess, and our ability to collate and compare that data, is variously hampered by:
- Heterogeneity and lack of structure in that data, both in terms of format and content;
- Accessibility problems due to lack of centralisation (siloing of data) and persistence of hard copy (as opposed to electronically stored) patient data;
- A lack of established reporting protocols to govern the terminology and standardise the data classifications used (eg, replacing the subjective use of terms such as “high” or “severe” with objective standards linked to clinical criteria); and
- A lack of templates to define and standardise the types of data collected, leading to gaps and lack of consistency in the data collected from one person to another.
At the WHF, Chisholm and Munir Pirmohamed, NHS chair of pharmacogenetics, described some of the efforts being made to address these data issues on a number of fronts, both process driven and technology driven:
- On the process front they discussed the need to develop the protocols, standards and templates that are currently lacking in clinical practice in a “genome-friendly” way.
- On the technology front they highlighted the need to apply novel methodologies like natural language processing, machine learning and artificial intelligence (AI) to the organisation and analysis of unstructured electronic health record data.
The role of AI in the processing and analysis of data was also highlighted at the WEF by Novartis CEO Vas Narasimhan, who observed that, as datasets increase in size and the range of possible parameters to be assessed and compared expands, AI will be able to analyse and detect signals in the data, or help to design trials, much more effectively than humans will be able to—provided that the quality data is there to train the AI.
Data privacy
Given the central importance of patient data, data privacy law also has an essential role to play in the establishment of the necessary framework for the secure collection and use of highly sensitive patient health and genomic data. This is especially the case in the current post-Facebook/Cambridge Analytica climate, in which the public has been sensitised to the significant risk of data privacy breaches and the consequences that can flow from them.
Without a secure framework, the risk of misuse of patient data would pose a real threat to precision medicine initiatives and potentially have a chilling effect on patient trust and buy-in. In particular, a serious data breach event at a sensitive stage in the adoption of precision medicine could set the project back significantly.
Data privacy laws in different jurisdictions impose different protective standards in relation to personal data, and particularly health, biometric and genetic data, which is typically treated as an especially sensitive category of data with higher levels of protection and restriction on use.
"These data regulation disparities pose real challenges to the collection of robust and comprehensive sets of patient data at scale."
These data regulation disparities pose real challenges to the collection of robust and comprehensive sets of patient data at scale, and to the sharing of that data across borders or with the industry partners that will be required to assume the risk of developing new treatments and diagnostics.
Cross-border transfer of personal data is restricted under many data protection regimes, and the standards and definitions of key concepts such as use, consent, anonymisation, data controllers, data processors and the scope of the application of data privacy laws will differ.
Notably, the EU General Data Protection Regulation, (GDPR), which harmonises data privacy laws across the EU (including the UK for the moment) and came into force on May 25, 2018, has extraterritorial effect, applying to any entity that processes the personal data of an EU data subject, regardless of its location.
It remains to be seen whether this extraterritorial scope has a positive harmonising effect globally or provokes countervailing extraterritorial regulation from other jurisdictions.
However, to the extent that the GDPR promotes a robust data privacy regime and increases harmonisation between different jurisdictions, this will probably be welcomed by stakeholders in precision medicine.
Conclusion
An understanding of the importance of data to the development of precision medicine approaches must be accompanied by an appreciation of the structural challenges that remain to be addressed in the secure and effective collection and use of that data.
The quality of outcomes from the precision medicine approaches to be developed will directly rely on the quantity, quality and diversity of the data on which they are based.
Having considered the role of data as an enabling prerequisite for precision medicine, the third instalment in this four-part series will focus on the practical challenges involved in translating research into clinical practice, particularly from the perspective of disruption to the traditional approach to medicine and clinicians trained according to that paradigm.
Daniel Lim is a partner at Kirkland & Ellis. He can be contacted at: daniel.lim@kirkland.com